How random is random?
Well-established methods for surrogate data fail to produce linear time series
Don’t trust too blindly to well-established methods, because they may have important but so far unknown pitfalls. This lesson was taught to scientists at the Max Planck Institute for Extraterrestrial Physics (MPE), when they had a closer look at two widely used algorithms for generating surrogates data. Surrogates are data sets that share some characteristics with the original data, while all other properties are subject to randomization. This method can be used, for example, to test for weak nonlinearities in a model-independent way, by comparing a real time series with surrogates that reproduce its linear properties.
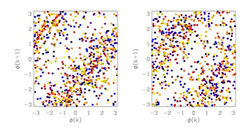
Since its introduction, the method of surrogates has found numerous applications in many fields of research ranging from geophysical and physiological time series analysis to econophysics, astrophysics, and cosmology. Researchers at MPE, in particular, use this method to analyse the cosmic microwave background radiation, an echo of the big bang. It is usually assumed that the small temperature fluctuations, which can be measured in this radiation, are distributed randomly in a Gaussian field. However, a careful analysis using surrogates revealed significant signatures for non-Gaussianities and asymmetries (see News 2011 - link).
In their recent work, the MPE scientists now put the method itself under scrutiny. They demonstrated that two commonly used algorithms for generating surrogates often fail to generate truly linear time series. Rather, the surrogates can show pronounced correlations, which should vanish for linear data.
The scientists applied their analysis to two quite distinct data sets: the first are X-ray observations of an active galaxy, where the light curves contain information about the physical processes in the innermost regions of these compact objects. The second data set are the day-to-day returns of the Dow Jones industrial index from 1896 to 2012. Both data sets are well suited for testing as they represent real data from a sufficiently complex system, where the mere detection of nonlinearities allows discriminating between models.
The analysis showed that for both data sets nonlinearities in the data remain undetected, if surrogates were produced using methods where phase correlations remain. This eventually leads to wrong physical or economical models for describing the data. A number of tests indicated that the presence of the phase correlations in this analysis is a generic property of the methods under study - rather independent of the underlying time series.
Thus, the scientists conclude that henceforth only surrogates should be used, for which the absence of nonlinearities is explicitly ensured. Otherwise intrinsically present nonlinearities might remain undetected and the wrong outcome of the surrogate test then leads to a wrong modeling of the complex underlying system.